layout:true
Introduction to Statistical Analysis
--- class: center, middle  # Introduction to Statistical Analysis - - - ## Instructors: Mark Yarish & Elizabeth DiLuzio ### Follow along at: http://bit.ly/intro-stats #### See the code at: http://bit.ly/intro-stats-code --- class:center,middle # Welcome ??? + Facilitators introduce themselves + Facilitators (respectfully) assert authority to be teaching material + Facilitators begin creating a safe, comfortable container for participants --- # A Few Ground Rules -- + Step up, step back -- + Be curious and ask questions! -- + Assume noble regard and positive intent -- + Respect multiple perspectives -- + Listen deeply -- + Be present (phone, email, social media, etc.) --- # Introductions and
Data Collection
+ Who are you? + Where do you work? + How many siblings do you have (not including yourself)? + How long have you worked for NYC in years? + What is your height (in inches)? ??? + Instructor uses [Google sheet to collect information](http://bit.ly/stats-data) as students introduce themselves --- # Goals for the Course -- + Review descriptive statistics in the context of operational decision making -- + Discuss correlation and simple linear regression analysis in the context of operational decision making -- + Introduce decision modeling and their use -- + Practice calculating descriptive statistics, calculating correlation, and developing predictive models in Excel --- # Key Takeaways for the Course -- + You will be more familiar with basic descriptive statistics -- + You will be better able to describe correlation and simple linear regression -- + You will better understand the value of decision models in operational decision making -- + You will be practiced in calculating descriptive statistics, calculating correlation, and developing predictive models in Excel --- # Key Assumptions -- + You’ve had some previous experience with statistics and probability -- + You’re familiar with using Excel to manipulate data and calculate values -- + You’re familiar with using formulas in Excel --- # Disclaimer -- + We're not statisticians -- + You won’t be a statistician by the end of this course -- + WeI often apply statistical tools and understanding in the work we do -- + We're assuming you all do the same, which is why you’re here --- # Housekeeping ??? + Facilitator sets expectations with the students + Establishes the "contract" for the class -- + We’ll have one 15 minute break in the morning -- + We’ll have an hour for lunch -- + We’ll have a 15 minute break in the afternoon -- + Class will start promptly after breaks -- + Feel free to use the bathroom if you need during class -- + Please take any phone conversations into the hall to not disrupt the class --- # Goals for this Morning -- + Review basic statistical measures -- + Practice using statistics in real-world applications -- + Familiarize you with how to use Excel for statistical analysis --- class:center,middle > We are drowning in information and starving for knowledge. ## John Naisbitt ??? + Facilitator prompts the participants to reflect on the value of statistics for understanding information + Helpful to make the distinction between the raw information and intelligence that can be used for decision making --- # Why Statistics? ??? + Facilitator emphasizes the utility of statistics for understanding information -- + Tools for extracting meaning from data -- + Commonly understood ways of communicating meaning to others ??? + Facilitator makes point about being able to compare using statistics + I often use the example of comparing one class with the other -> if mean years of service is higher than this class, what might that imply about the --- class:center,middle # Let’s run the statistics on our class today ## Download
the data for the class
 ??? + Facilitator leads the participants through applying basic descriptive stats to the data collected at the introductions + Facilitator reviews each concept with participants then leads them through calculating it in the appropriate place on the spreadsheet --- # Mean -- + A representative value for the data -- + Usually what people mean by “average” -- + Calculate by adding all the values together and dividing by the number instances -- + Sensitive to extremes -- ## .dp-orange[Calculate the means (number of siblings, years of service, and height) for our class today] --- # Median -- + The “middle” value of a data set -- + Center value of a data set with an odd number of values -- + Sum of two middle values divided by 2 if the number of items in a data set is even -- + Resistant to extreme values --  -- ## .dp-orange[Calculate the medians for our class today] --- exclude:true # Median vs Mean  --- # Mode  -- + The most frequent value in a dataset -- + Often used for categorical data -- ####
## .dp-orange[Calculate the mode for our class today] --- # Median vs Mean vs Mode  By Cmglee (Own work)
CC BY-SA 3.0
or
GFDL
,
via Wikimedia Commons
??? + Facilitator describes the difference between mean, median, and mode, emphasizing when you would use one over the other --- # When Do We Use Median rather than Mean (Average)? ??? + Facilitator reflects with participants when they recall using median rather than mean in their work or in the media + Establishes key learning point of when these measure should be used (depends on the shape of the data) -- + House Prices -- + Household Income -- + What else? -- + Why? --- # Anscombe's Quartet  ??? + Facilitator uses example of Anscombe's Quartet to demonstrate the need to visually inspect data + For more information, see [this article](https://heap.io/blog/data-stories/anscombes-quartet-and-why-summary-statistics-dont-tell-the-whole-story) --- # Histogram --  + Charts the frequency of instances in the data -- + Shows the frequency distribution -- + Values are grouped into class intervals --  + Best to have a consistent size to class intervals --
.caption[http://mathematica.stackexchange.com/questions/59520/histogram-with-variable-bin-size] --- class:center,middle # Creating a Histogram for Height in Post-Its ??? +Have participants write their height, in inches, on a post-it +Collect and graph post-its on poster paper +Point out that there are a lot of bars that are quite small +Not very helpful in understanding trends in our data +What happens when we group the bars together? Group evenly to be sure we can draw conclusions. +Can you see a trend better now? --- class:center,middle # Creating a Histogram for Height in Excel --- # Installing Data Analysis ToolPak  + File + Options + Add-ins + Manage + “Go…” --- # Installing Data Analysis ToolPak  --- # Setup Your Bins ??? + Starting with arbitrarily determining bin size by range helps to introduce the topic + Later introduce equal interval based on either range of the data or the limits of the lower and upper bounds to eliminate outlines + Key learning point is that we control how to tell the story of the data with the bin size we select + Easily to obscure or manufacture a pattern to the data by picking the wrong bin size --  + Use an empty column and label it “Bins” -- + Start with the max of the first bin -- + Create an entry for each bin you want -- + Use a formula to save time --- # Creating a Histogram (Height) ## .center[Under the Data Ribbon]  --- # Creating a Histogram (Height)  --- # Creating a Histogram (Height)  --- # Creating a Histogram (Height)  --- class:center,middle # Distributions of Data --- # Normal(-ish) Distribution  --- # Long-tail Distribution  --- # Bi-Modal Distribution  --- # Measures of Central Tendency -- + Quantitative data tends to cluster around some central value -- + Contrasts with the spread of data around that center (i.e. the variability in the data) -- + Mean is a more precise measure and more often used -- + Median is better when there are extreme outliers -- + Mode is used when the data is categorical (as opposed to numeric) --- class:center,middle # Measuring Variability --- # Range -- + The gap between the minimum value and the maximum value -- + Calculated by subtracting the minimum from the maximum -- + Use the [`MAX`](https://support.office.com/en-us/article/MAX-function-e0012414-9ac8-4b34-9a47-73e662c08098) and [`MIN`](https://support.office.com/en-us/article/MIN-function-61635d12-920f-4ce2-a70f-96f202dcc152) functions in Excel to calculate this for our data --- # Quartiles ??? + Facilitator introduces concept by discussing percentiles + Prompts participants with the scenario: "Your child comes home and says they scored on the 98th percentile on the SAT. What does that mean?" + The answer is that they scored at or above 98% of the students who took the SAT + This introduces the idea important to understanding Quartiles as breaking up the data into 4 equal portions of the data --  .caption-small[[Image](https://commons.wikimedia.org/wiki/File:Iqr.png) credit Ark0n [CC BY-SA 3.0](http://creativecommons.org/licenses/by-sa/3.0/)] -- + Quartiles split the data into four equal groups -- + First quartile is 0-25% of the data -- + Second quartile is 25-50% of the data -- + Third quartile is 50-75% of the data -- + Fourth quartile is 75-100% of the data -- + Use the [`QUARTILE`](https://support.office.com/en-us/article/QUARTILE-function-93cf8f62-60cd-4fdb-8a92-8451041e1a2a) function in Excel to calculate this --- # Interquartile Range -- + “Middle” 50% of data (between 1st Quartile and 3rd Quartile) --  .caption[[Image source](https://community.qlik.com/t5/Qlik-Design-Blog/Recipe-for-a-Box-Plot/ba-p/1471745?)] --- # Outliers  -- + Any data points less than 1.5x the IQR or greater than 1.5x the IQR are considered outliers -- + Helps identify data points that may skew the analysis -- + Focus on the “meat” of the data -- # .caption-right[[Image source FlowingData.com](http://flowingdata.com/2008/02/15/how-to-read-and-use-a-box-and-whisker-plot/)] --- name:calc-iqr class:center,middle # Do We Have Any Outliers in Our Data? ??? + First calculate the Upper Limit -> participants will usually calculate the formula as `=1.5 * IQR + Q3` + In calculating the Lower Limit -> participants will use the same order to get `=1.5 * IQR - Q1`, which leads to an incorrect result. Make sure to point this out to them + Reflect on any outliers with the class --- # Standard Deviation -- + The average distance of each data point from the mean --  -- + Larger the standard deviation, the greater the spread --  --- # Standard Deviation  ??? + Facilitator discusses the idea of standard deviations and the amount of data at each deviation + For more information, see: https://simple.wikipedia.org/wiki/Standard_deviation + For the breakdowns of standard deviations, see https://www.robertniles.com/stats/stdev.shtml --- # Measures of Variability -- + Describe the distribution of our data -- + Range (Maximum – Minimum) -- + Inter-quartile Range -- + Standard Deviation -- + Identification of outliers (1.5 x IQR) --- # Descriptive Statistics -- + Quantitatively describe the main features of a dataset -- + Help distinguish distributions and make them comparable -- + 5 number summary --
- Minimum --
- 1st Quartile --
- Median --
- 3rd Quartile --
- Maximum --- # Exploratory Data Analysis -- + Goal -> Discover patterns in the data -- + Understand the context -- + Summarize fields -- + Use graphical representations of the data -- + Explore outliers -- ### Tukey, J.W. (1977). Exploratory data analysis. Reading, MA: Addison-Wesley. --- class:center,middle # 15 MIN BREAK 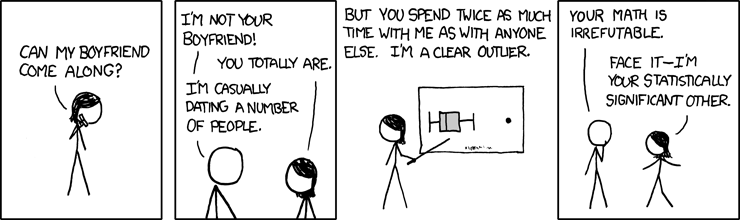 Source: https://xkcd.com/539/ --- class:center,middle # Let's Try That Again # [Vehicle Collisions in NYC](data/20160401_0630_NYPD_Motor_Vehicle_Collisions.xlsx) --- class:center,middle # But before we get too deep into data... ??? + Facilitator demonstrates the concepts of a pivot table by doing a human pivot table + Facilitator asks participants to move to the front of the room + Facilitator leads them through exercises to show sort, filter, and aggregate --- class:center,middle  --- # PivotTables ??? + Facilitator introduces concept and usage of pivottables to the class + Best to introduce by having someone describe how they use a pivottable in their work (connects to the day-to-day concretely) -- + A data summarization tool -- + Useful to quickly understand data -- + Can use to graph data totals --  --- # Creating a PivotTable  ??? + Facilitator describes the steps to creating a pivottable in Excel + Please don't model selecting all data before selecting "Insert Pivottable" -> creates `(blank)` field in pivottable + Just allow to select all data by itself -- + Should default to all your data .red[unless you have any cells selected] ??? + Remind participants not to have any data selected when inserting a pivottable. When this comes up in class (because someone did it), use it as a teachable moment -- + Should default to a new worksheet --- # Creating a PivotTable  ## Drag and drop fields to visualize -- + Row labels -- + Values -- + Filter -- + Column Labels --- # Creating a PivotTable of Dates  --- exclude:true # Creating a PivotTable  --- # Calculating Descriptive Statistics  --- # Calculating Descriptive Statistics  --- # Calculating Descriptive Statistics  --- # Calculating Descriptive Statistics  --- # Questions of the Data -- + What is the mean number of accidents per day? -- + Is mean or median the best way to describe this data? -- + Are there any outliers in this data? --- # Wrap-Up ??? + Facilitator reviews the concepts introduced in the morning and ensures all questions are answered -- + Reviewed basic descriptive statistics -- + Calculated basic descriptive statistics in Excel -- + Discussed histograms -- + Created histograms in Excel -- + Analyzed NYC motor vehicle collision data --- class:center,middle # Lunch --- class:center,middle # Welcome Back! --- class:center,middle # Let's Get Back to the Data ## What is the mean (average) time a [311 noise-related service request](data/20140601_20140901_311_noise.csv) remains open? The median? ??? + Facilitator allows participants to apply learning in a release exercise --- # Preparing the Data - Insert Column  --- # Preparing the Data - Calculate Days Open  --- # Preparing the Data - Format Result  --- # Preparing the Data - Calculate Hours Open  --- class:center,middle # Now Let's Calculate the Descriptive Statistics --- # Calculating Descriptive Statistics  --- # Calculating Descriptive Statistics  --- # Calculating Descriptive Statistics  -- + What's missing? -- + Q1 -- + Q3 -- + IQR -- + Upper Bound -- + Lower Bound -- ## .dp-orange[Calculate those now
(trust us, it'll be useful)] --- # Creating a Histogram  --- # Creating a Histogram  --- # Creating a Histogram - Bin Size 100  --- # Creating a Histogram - Bin Size 100  --- # Creating a Histogram - Bin Size 50  --- # Creating a Histogram - Bin Size 50  --- # Creating a Histogram - Formatting Histogram  --- # Creating a Histogram  --- class:center,middle # Do these tell a true and compelling story? # --- class:center,middle # Do these tell a true and compelling story? # What do we do about that? --- # Things to Think About -- + Do we need to display all of the data? -- + What data do we keep? -- + How do we determine what to show? -- + How do we be clear about what we're not showing? --- # Removing Outliers (Using 1.5 x IQR)  --- # Removing Outliers (Using 1.5 x IQR) --  + Creating 10 equal bins (IQR of 9.275 divided by 10) -- + Alternative strategy for determining bins --- # Removing Outliers (Using 1.5 x IQR) --  + Only 3,659 service requests greater than 9.275 hours (upper bound) -- + Represents less than 10% (~9.73%) of 37,615 total service requests --- # What Do We Know? -- + The median time a noise complaint is open is 2 hours -- + 50% of the noise complaints are closed between 1-4 hours (median is 2 hours, IQR is 3 hours) -- + There is a long tail of complaints that take longer to close (range of 1158 hours, standard deviation of 36 hours) --- class:center,middle # 15 Min Break 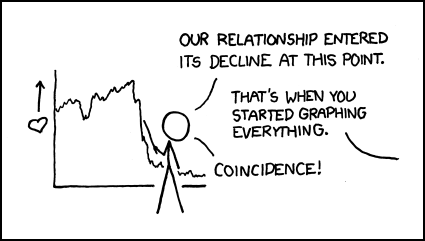 [Source](https://xkcd.com/523/) --- # Correlations -- + Values tend to have a relationship -- + That relationship can be of several types --
- Proportional (increase in one increases the other) --
- Inversely proportional (increase in one decreases the other) -- + Example -> Height and weight --- # Correlations - Height and Weight  ### How do we measure this relationship? --- # Coefficient of Correlation ??? + Facilitator provides examples of r values with their corresponding visualizations -- + Quantifies the amount of shared variability between variables -- + Ranges between -1 and +1 --
- Negative numbers are inversely proportional --
- Positive numbers are directly proportional --
- The closer to either -1 or +1, the greater the correlation --- # Coefficient of Correlation  http://www.statisticshowto.com/what-is-the-correlation-coefficient-formula/ --- # Coefficient of Correlation  http://pixshark.com/correlation-examples.htm ---  ??? + Facilitator provides example of situation where correlation is high because the variables aren't independent (flight departure delay and flight arrival delay) --- # Correlations - Height and Weight  ## [Download the data so we can check this out](https://github.com/datapolitan/CenterForGovernmentExcellence/blob/gh-pages/data/height_weight.xlsx?raw=true) --- # We can use the formula...  --- # ...Or we could use Excel  --- class:center,middle # [Let's Try That Again](data/2013_NYC_CD_MedianIncome_Recycle.xlsx) ??? + Recycling Rate is based on the measurements DSNY takes of its trucks entering and leaving the dump facility (called "tipping") -> A truck is weigned on the way in to tip and on the way out. The difference is the amount tipped + The recycling rate is the weight of recyclables / the total weight of collected refuse + Sanitation districts align with community districts -> trucks collect only in that CD and the CD is logged by the truck --- # Correlations - Recycling and Median Income  ### These are even slightly more correlated (r=0.88478) [Check it yourself](https://github.com/datapolitan/CenterForGovernmentExcellence/blob/gh-pages/data/2013_NYC_CD_MedianIncome_Recycle.xlsx?raw=true) http://iquantny.tumblr.com/post/79846201258/the-huge-correlation-between-median-income-and --- # Correlations - Recycling and Median Income  --- # Coefficient of Determination -- + The percentage of variance in one variable shared with the other -- + More shared variability implies a stronger relationship -- + Calculate by squaring the correlation coefficient --
- Ex. The correlation of determination for median income vs recycling rates is 78% --- class:center,middle # However... --- # Correlations  [Source](http://tylervigen.com/view_correlation?id=1703) ??? + Facilitator provides example of spurious correlation to prompt a discussion about the difference between correlation and causation + Great question to ask: "Do you think the consumption of margarine has anything to do with the divorce rate in Maine?" -> Important to point out the R value is very high + Lots of opportunity for coincidental correlations to occur and we should be aware of that --- # Correlations  ??? + Facilitator discusses the first case (`X->Y`) as the ideal, but then introduces the other situations that are more likely in the real world + A good example of this is the correlation between [ice cream sales and homicides](https://slate.com/news-and-politics/2013/07/warm-weather-homicide-rates-when-ice-cream-sales-rise-homicides-rise-coincidence.html) -- ## Correlation does not imply causation ??? + Describe the [Randomized Controlled Trial](https://himmelfarb.gwu.edu/tutorials/studydesign101/rcts.cfm) and prompt participants to reflect on whether they have enough control over their data to make causal inferences --- class:middle,center # Predictive Modeling ??? + After establishing that we can't draw causal inferences, we can use the correlations to make predictions about data we don't know based on what we do know + Facilitator emphasizes that models are only as good as the data and understanding you have of what the data says --- # Prediction -- + Knowing the relationship between variables (i.e. the correlation), we can predict values based on the relationship -- + Can estimate the magnitude as well as the general trend -- + More data points, the better the prediction -- + Example -> Knowing the relationship between median income and recycling rates, what can we predict about recycling rates as median incomes grow in communities? --- # Linear Regression -- + Using the known relationship between continuous variables, we can predict unseen values -- + Assumes relationship is linear ??? + Great example of this is the work MODA did in support of the initial roll-out of Pre-K for All when the city needed to find space quickly for centers + MODA compared the utility usage with the square footage of city buildings -> utility usage and occupancy is a linear relationship, with each person added to an area consuming a roughly even amount of utilities + outliers with high square footage and low utility usage were likely under-utilized and could potentially be available to be used --- # Formula for a line  ##### http://www.algebra-class.com/slope-formula.html --- # Formula for a line  ##### http://www.mathwarehouse.com/algebra/linear_equation/slope-of-a-line.php --- # Formula for a line -- + Draw a line that minimizes the distance between each point -- + “Line of best fit” -> minimizes the sum of squared residuals --  ##### http://nbviewer.ipython.org/github/justmarkham/DAT4/blob/master/notebooks/08_linear_regression.ipynb --- # Linear Regression -- + Characteristics of the line defines the relationship -- + Slope -> relationship between independent and dependent variable (how Y increases per unit of X) -- + Intercept -> expected mean value of Y at X=0 -- + Values along the line are the predicted values for any given value X --- # Displaying a Trendline in Excel  --  --- # Calculating coefficients in Excel  --- # Calculating coefficients in Excel  --- # Calculating coefficients in Excel  --- # Linear Regression Line  -- ### RecyclingRate = 0.0000001869 * MedianIncome + 0.07480414 --- # What is this telling us? ### RecyclingRate = 0.0000001869 * MedianIncome + 0.07480414 -- + Every dollar increase in median income will increase the recycling rate by about 0.0000001869 -- + If the median income was zero, the recycling rate would be about 0.07480414 -- + What is the expected recycling rate for a community district with a median Household income of $70,000? -- `0.000001869 * 70000 + 0.07480414 = 0.205677023` --- class:center,middle # We've created a model to make predictions! ## # --- class:center,middle # We've created a model to make predictions! ## # The predictions are just not very good --- class:center,middle  --- exclude:true class:center,middle  --- # How is Linear Regression Useful in Cities? -- + Make predictions -- + Identify outliers --- # Making Decisions in a Resource Constrained World ??? + Facilitator invites participants to reflect on whether they have enough resources for the work they do (they don't) + Facilitator invites them to reflect on what they often come up short on -- + Types of constraints --
- Money --
- Time --
- Resources --
- Political Concerns -- + Need ways to optimize around what’s available --- # Decision Modeling -- + The use of mathematical or scientific methods to determine allocation of time, money, and/or other resources -- + Meant to improve or optimize the performance of a system -- + Other terms: --
- Operations research --
- Management science --- # Decision Modeling Process  ??? + Facilitator describes an example real-world system or process that exists for which we're trying to optimize --- # Decision Modeling Process  ??? + Facilitator describes the process of modeling that system, however imperfectly into a model of how that system or process works --- # Decision Modeling Process  ??? + Facilitator describes the means by which we model the system is by setting up a series of equations and inequalities + These represent how the system works (how many of X can be processed at a time, how many Y hours it takes to produce a given amount, etc.) --- # Decision Modeling Process  ??? + From manipulating these equations and inequalities, we arrive at some conclusions about how the system works and can be optimized --- # Decision Modeling Process  ??? + We then make some correlation between the model and the real world system: how does this impact how we manage our resources in the real world? + Examples include staffing, expenditure, or other allocation of resources --- # Decision Modeling Process  ??? + The final step is to implement the conclusions in the real world + Because our model is only an approximation, then we need to assess the real-world impact + Making a change in the "real world" will also impact our models, as they need to change to reflect the new way in which the system operates --- # Requirements of DM Process -- + You have to understand the real world process -- + You have to be able to quantify the real world process -- + You need to test your assumptions -- + The decisions made based on the model will have an impact that need to be accounted for in the future --- class:center,middle # Optimizing Parking Ticket Revenue # Given an understanding of the basic constraints at work, we can optimize the placement of ticket agents around NYC to maximize revenue --- # Constraints -- + Density of illegally parked vehicles varies by location -- + Number of illegally parked vehicles varies by location -- + Amount of fine varies by location -- + Number of agents is limited -- + Only so many tickets an agent can write in a day -- + Only so many tickets are actually paid -- + Some neighborhoods are more concerned about illegal parking than others -- + Every borough must have at least one ticket agent --- # Constraints  --- class:center,middle # Now let’s optimize!  .caption[Photo by [Jungwoo Hong](https://unsplash.com/photos/cYUMaCqMYvI?utm_source=unsplash&utm_medium=referral&utm_content=creditCopyText) on [Unsplash](https://unsplash.com/search/photos/improvement?utm_source=unsplash&utm_medium=referral&utm_content=creditCopyText)] ## Click to [open our practice dataset](data/decision_model.xlsx) --- # Setup Spreadsheet  --- # Sum Assigned Agents  --- # Add Total Number of Agents (1000)  --- # Sum Revenue  --- class: center,middle # Now for those systems of equations...
via GIPHY
--- # Calculating Number of Tickets -- + Number of Agents -- + Multiplied by the number of tickets they can write (200) -- + Multiplied by the density of illegally parked cars -- ``` Number of Tickets = # of Agents * 200 * Density of Illegally Parked Cars ``` --- # Calculating Number of Tickets - Example ``` Number of Tickets = # of Agents * 200 * Density of Illegally Parked Cars ``` -- + 100 Agents in Manhattan -- + Max number of tickets an agent can write is 200 -- + The density of illegally parked cars is 40% -- ``` Estimated Number of Tickets = 100 * 200 * 0.4 = 8,000 ``` --- # Add Ticketing Calculation ``` Ticketed = # of Agents * 200 * Density of Illegally Parked Cars ```  --- # Calculating Revenue -- + Number of Agents -- + Multiplied by the number of tickets they can write (200) -- + Multiplied by the fine in that borough -- + Multiplied by the percentage of fines collected in that borough -- + Multiplied by the density of illegally parked cars -- ``` Revenue = # of Agents * 200 * Fine * % Collect * Density of Illegally Parked Cars ``` --- # Calculating Revenue - Example ``` Revenue = # of Agents * 200 * Fine * % Collect * Density of Illegally Parked Cars ``` -- + 100 Agents in Manhattan -- + Max number of tickets an agent can write is 200 -- + Fine is $90 -- + They collect 75% of fines -- + The density of illegally parked cars is 40% -- ``` Estimated Revenue = 100 * 200 * 90 * 0.75 * 0.4 = $540,000 ``` --- # Add Revenue Calculation ``` Revenue = # of Agents * 200 * Fine * % Collect * Density of Illegally Parked Cars ```  --- # Spreadsheet Setup  ## Everything is now setup as a set of relationships (equations) --- # Excel will find optimal number of agents  --- # Installing Solver  + File + Options + Add-ins + Manage + “Go…” --- # Installing Solver  --- # Using Solver  --- # Using Solver - Parameters Dialog Box  --- # Using Solver - Setting Objective  --- # Using Solver - Setting Objective  + Maximize the value in cell `Sheet1!$I$9` (Total Revenue)  --- # Using Solver - Cells to be Changed  -- + Tell Excel the cells to change to reach the objective  --- # Constraints -- + Number of assigned agents must be greater than or equal to minimum but less than or equal to the maximum -- + The number of tickets can’t exceed the estimated number of illegally parked cars -- + The total number of assigned agents must be less than or equal to 1000 --- # Constraints + Number of assigned agents must be greater than or equal to minimum but less than or equal to the maximum  --- # Constraints + The number of tickets can’t exceed the estimated number of illegally parked cars  --- # Constraints + The total number of assigned agents must be less than or equal to 1000  --- # Final Touches --  + Set solving method to `Simplex LP` --  + Check over parameters and then click `Solve` --- # Results  -- + This is the optimal assignment of ticket agents to maximize revenue -- + We can adjust the parameters to test different conditions -- + Averaging the results of different models helps us arrive at a more reliable estimate --- exclude:true # Results + If you like the results, click `OK`  + Otherwise you can go back to adjust parameters --- class:center,middle # Wrap-Up 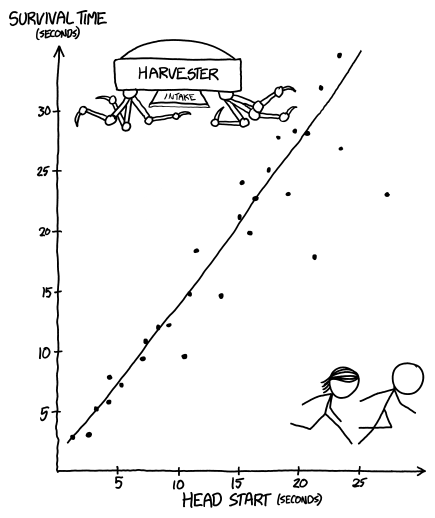 .caption[[Source](https://what-if.xkcd.com/120/)] --- # Goals for the Course -- + Review descriptive statistics in the context of operational decision making -- + Discuss correlation and simple linear regression analysis in the context of operational decision making -- + Introduce decision modeling and their use -- + Practice calculating descriptive statistics, calculating correlation, and developing predictive models in Excel --- # Key Takeaways for the Course -- + You will be more familiar with basic descriptive statistics -- + You will be better able to describe correlation and simple linear regression -- + You will better understand the value of decision models in operational decision making -- + You will be practiced in calculating descriptive statistics, calculating correlation, and developing predictive models in Excel --- # Datasets for Class + [Class data](http://bit.ly/stats-data) + [Vehicle Collision Data](data/20160401_0630_NYPD_Motor_Vehicle_Collisions.xlsx) + [311 Noise Complaint Data](data/20140601_20140901_311_noise.csv) + [2013 Recycling Data](data/2013_NYC_CD_MedianIncome_Recycle.xlsx) + [Height and Weight data](data/height_weight.xlsx) + [Decision Model data](data/decision_model.xlsx) --- # Key Excel Functions for Statistics + [`=AVERAGE()`](https://support.office.com/en-us/article/AVERAGE-function-047bac88-d466-426c-a32b-8f33eb960cf6): Calculates the arithmetic mean for a range of numbers + [`=MEDIAN()`](https://support.office.com/en-us/article/MEDIAN-function-d0916313-4753-414c-8537-ce85bdd967d2): Calculates the median for a range of numbers + [`=MODE()`](https://support.office.com/en-us/article/MODE-function-e45192ce-9122-4980-82ed-4bdc34973120): Calculates the mode for a range of numbers + [`=MAX()`](https://support.office.com/en-us/article/MAX-function-e0012414-9ac8-4b34-9a47-73e662c08098) and [`=MIN()`](https://support.office.com/en-us/article/MIN-function-61635d12-920f-4ce2-a70f-96f202dcc152): Calculates the maximum and minimum number for a range of numbers + [`=QUARTILE()`](https://support.office.com/en-us/article/QUARTILE-function-93cf8f62-60cd-4fdb-8a92-8451041e1a2a): Calculate quartiles for a range of numbers + [`=CORREL()`](https://support.office.com/en-us/article/CORREL-function-995dcef7-0c0a-4bed-a3fb-239d7b68ca92): Calculates the coefficient of correlation between two ranges of numbers --- # Have trouble with the analysis? + [Height and weight correlations](data/height_weight_processed.xlsx) + [Median income and recycling data processed](data/2013_NYC_CD_MedianIncome_Recycle_processed.xlsx) + [Decision model completed](data/decision_model_complete.xlsx) + [Complete data download](data/intro_stats_data.zip) --- # For More Information -- + [Statistics for People Who (Think) They Hate Statistics](https://us.sagepub.com/en-us/nam/statistics-for-people-who-think-they-hate-statistics/book246898) -- + [Data Smart: Using Data Science to Transform Information into Insight](https://www.wiley.com/en-us/Data+Smart%3A+Using+Data+Science+to+Transform+Information+into+Insight-p-9781118661468) -- + [IQuantNY blogpost "The Huge Correlation between Median Income and Recycling in NYC"](http://iquantny.tumblr.com/post/79846201258/the-huge-correlation-between-median-income-and) -- + [Copy of Workbook for Class Today](workbook.pdf) -- + [Datapolitan Training Classes](http://training.datapolitan.com) --- # .center[Contact Information] ## Mark Yarish + Email: mark[at]datapolitan[dot]com + Twitter: [@MarkYarish](https://twitter.com/MarkYarish) ## Elizabeth DiLuzio + Email: elizabeth[at]datapolitan[dot]com + Twitter: [@LizDiLuzio](https://twitter.com/lizdiluzio) --- class:center,middle # Thank You!